In boardrooms across industries, a familiar scenario plays out with alarming frequency: executives review promising AI pilot results, only to find themselves six months later questioning why these initiatives haven’t scaled beyond their initial scope. This phenomenon, aptly termed “pilot purgatory,” has become the graveyard of digital transformation aspirations for many organizations. According to recent McKinsey research, while 56% of companies report adopting AI in at least one function, only 27% report successful deployment at scale. Meanwhile, organizations that successfully transition from pilots to production report an average 20-30% increase in productivity and a 15-20% reduction in operational costs within the first year of scaled implementation.
The gap between experimental success and enterprise-wide value realization represents more than just a technical challenge – it signals a fundamental disconnect between how organizations approach AI pilots versus how they manage production systems. This blog explores the critical barriers to AI scaling and provides a pragmatic roadmap for transitioning from promising pilots to production systems that deliver measurable business value.
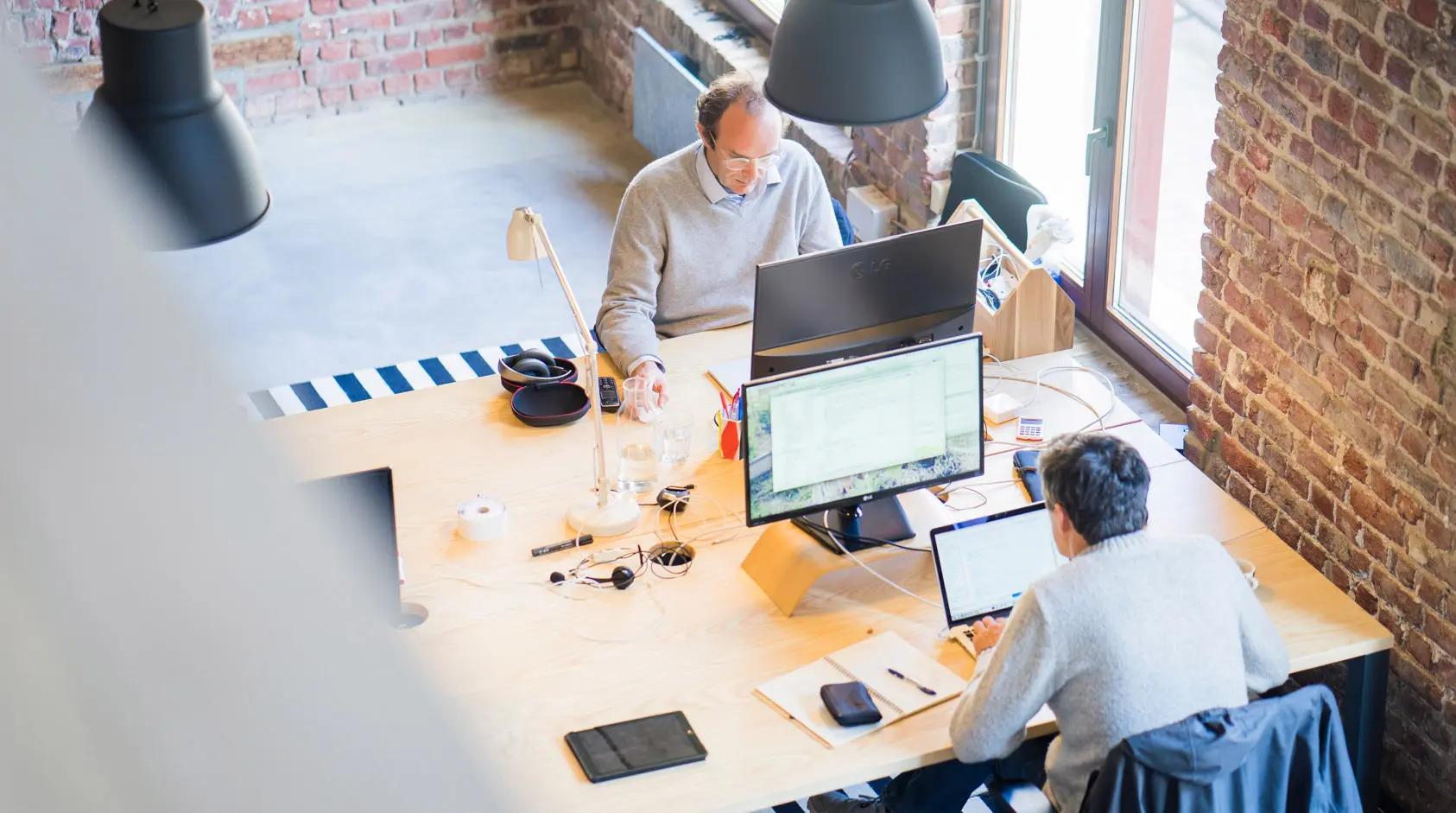
The Pilot Purgatory Phenomenon: Understanding the Gap
The journey from AI experimentation to enterprise-scale implementation is fraught with challenges that extend far beyond the technical performance of models. Understanding these barriers is the first step toward overcoming them
Common Barriers to Scaling
Misaligned Success Metrics: Pilot projects often prioritize technical performance (accuracy, precision, recall) over business-oriented metrics (cost reduction, revenue impact, customer satisfaction). This disconnect creates the illusion of success while failing to demonstrate tangible business value.
Insufficient Infrastructure Planning: Many AI pilots operate in isolated environments with curated data, ideal conditions, and limited scope. Scaling requires confronting the messy reality of enterprise infrastructure, data pipelines, and integration requirements – challenges rarely addressed during the pilot phase.
Governance and Risk Oversight Gaps: As AI systems scale, their impact and associated risks grow exponentially. Organizations frequently underestimate the need for robust governance frameworks, risk assessment processes, and ethical considerations that become critical at scale.
Skills and Organizational Readiness: The technical skills required to build a successful pilot differ substantially from those needed to operate production AI systems. Many organizations lack the DataOps, MLOps, and AI engineering capabilities essential for scaling.
Failure to Address Change Management: Perhaps most critically, organizations often treat AI implementation as a purely technical challenge, neglecting the organizational change management required for successful adoption.
A study by MIT Sloan Management Review found that among failed AI scaling efforts, 65% attributed the failure to organizational and people-related challenges rather than technical limitations. This underscores a fundamental truth: scaling AI is as much an organizational transformation as it is a technological one.
"The gap between experimental success and enterprise-wide value realization represents more than just a technical challenge - it signals a fundamental disconnect between how organizations approach AI pilots versus how they manage production systems."
The Scaling Roadmap: From Pilot to Production
Successfully transitioning from pilots to production requires a structured approach that addresses both technical and organizational dimensions. Here’s a comprehensive roadmap to navigate this critical journey:
Phase 1: Strategic Alignment and Foundation Building
Business Value Definition: Before scaling any AI initiative, rigorously define and validate the business case. Establish clear KPIs that align with strategic priorities and ensure stakeholder agreement on how success will be measured.
Example: A major retail bank shifted from measuring their fraud detection model’s accuracy to measuring reduction in false positives and the resulting operational cost savings from fewer manual reviews. This reframing helped secure executive buy-in for scaling the solution across multiple product lines.
Technical Debt Assessment: Conduct a thorough evaluation of existing systems, data infrastructure, and technical capabilities. Identify integration requirements, potential bottlenecks, and infrastructure needs before scaling begins.
Governance Framework Development: Establish a governance structure that addresses model development standards, monitoring requirements, risk thresholds, and ethical considerations. This framework should evolve from light guardrails during piloting to comprehensive oversight for production.
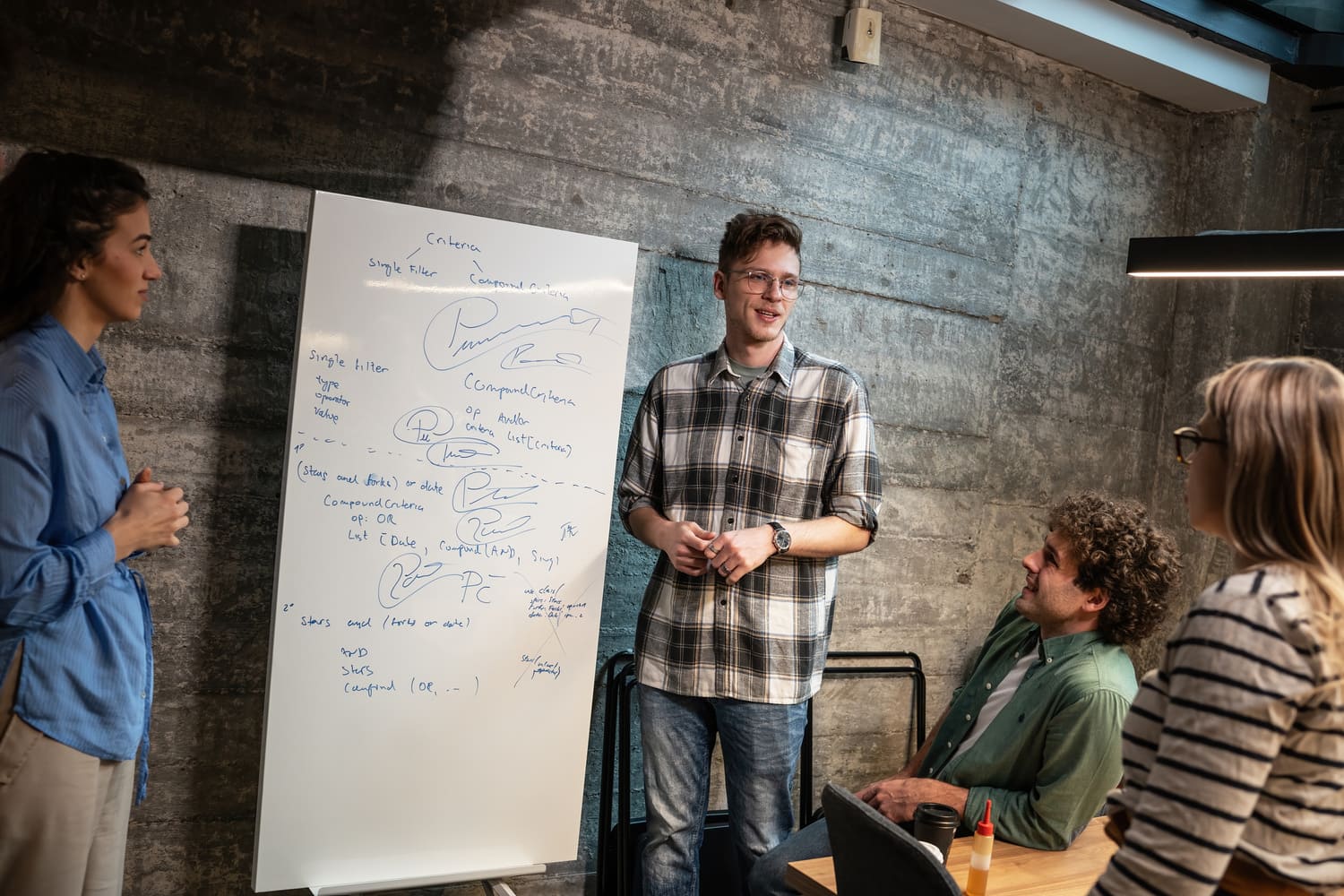
Phase 2: Architectural and Operational Readiness
Scalable Architecture Design: Transition from pilot architectures (often optimized for flexibility and speed) to production architectures designed for reliability, security, and performance at scale. Consider:
- Data pipeline resilience and throughput
- Model serving infrastructure
- Integration with existing enterprise systems
- Monitoring and observability capabilities
MLOps Implementation: Develop the operational backbone for AI at scale, including:
- Continuous integration/continuous deployment (CI/CD) pipelines for model updates
- Automated testing frameworks
- Model versioning and registry systems
- Feature stores for consistent data access
- Monitoring systems for model drift and performance
Example: A healthcare provider implemented a feature store that standardized how patient risk factors were calculated across multiple AI models. This not only improved model maintainability but reduced regulatory compliance overhead by centralizing data transformation logic.
Skills Development and Team Structure: Assess capability gaps and develop a talent strategy that addresses the full spectrum of needs for AI at scale:
- Data engineering
- MLOps and DataOps
- Model risk management
- AI product management
- Change management expertise
Phase 3: Scaled Implementation and Value Realization
Phased Rollout Strategy: Develop a structured approach to scaling that includes:
- Pilot expansion to adjacent use cases
- Parallel implementation across multiple business units
- Progressive feature releases to manage risk
- Performance benchmarking at each stage
Change Management and Adoption: Implement a comprehensive change management program that addresses:
- Stakeholder communication and expectation setting
- Training and capability building for end-users
- Process redesign to maximize AI impact
- Continuous feedback loops for improvement
Value Tracking and Optimization: Establish mechanisms to measure, report, and optimize business value throughout the scaling process:
- Regular value assessment reviews
- Feedback mechanisms from business users
- Continuous improvement processes
- Executive dashboards for visibility
Example: A manufacturing organization implemented a “value tracking war room” that met weekly during their predictive maintenance AI scaling. This cross-functional team tracked KPIs, addressed adoption barriers, and continuously refined the implementation approach, ultimately achieving 3x the projected ROI.
"Scaling AI is as much an organizational transformation as it is a technological one. The organizations that successfully navigate this transition approach AI scaling as a strategic business initiative rather than a technology project."
Industry Implementation Examples
The principles outlined above take different forms across industries. Here are examples of successful scaling approaches in three sectors:
Banking and Financial Services
A global bank successfully scaled their customer churn prediction models from a single product line to enterprise-wide implementation by:
- Creating a centralized feature store for customer attributes
- Developing standardized model monitoring dashboards
- Implementing a tiered rollout strategy based on product profitability
- Establishing cross-functional “AI adoption squads” combining data scientists, IT specialists, and business stakeholders
The results included a 34% reduction in high-value customer attrition and an estimated $156M in preserved annual revenue.
Healthcare
A healthcare network scaled their patient readmission prediction system by:
- Building an integrated data pipeline connecting their EHR system with their AI platform
- Creating standard interfaces for model outputs to be consumed by clinical systems
- Developing clinician-specific training programs to build trust in AI recommendations
- Implementing a robust ethics review process for each expansion phase
This approach led to a 27% reduction in preventable readmissions and approximately $42M in annual savings from improved resource allocation.
Manufacturing
A global manufacturer successfully scaled their predictive maintenance solution across multiple factories by:
- Standardizing sensor data collection and preprocessing
- Creating modular model architectures that could be customized for different equipment types
- Developing shop floor visualization tools that integrated with existing workflows
- Implementing a train-the-trainer program for maintenance teams
The scaled implementation resulted in a 31% reduction in unplanned downtime and a 24% extension in equipment lifespan.
Business Impact and ROI Considerations
Organizations that successfully scale AI initiatives report significantly higher returns than those stuck in pilot purgatory. According to Deloitte’s State of AI in the Enterprise survey, companies with multiple production AI systems report:
- 2.5x higher revenue impact from AI initiatives
- 3x greater cost reduction
- 4x higher rates of process improvement
- Substantially higher workforce productivity
However, these benefits come with investment requirements that must be carefully planned:
Infrastructure Costs: Scaling requires robust data pipelines, computing resources, and operational tools. Cloud-based implementations can help manage these costs but require careful architecture planning.
Talent Investment: Organizations typically underestimate the skills required for scaled AI. Successful implementations often involve a mixture of hiring, upskilling, and strategic partnerships.
Process Adaptation Costs: Realizing AI’s full value often requires process redesign and organizational adaptation, which carry both direct and indirect costs.
Governance and Compliance: As AI scales, so do the resources required for responsible implementation, particularly in regulated industries.
The most successful organizations view these not as costs but as investments in building lasting competitive advantage through AI capabilities.
"As AI continues to transform industries, the ability to move beyond isolated experiments to scaled implementation will increasingly separate market leaders from laggards."
Conclusion: Breaking Free from Pilot Purgatory
The journey from AI pilots to production is challenging but increasingly necessary for organizations seeking to remain competitive. The organizations that successfully navigate this transition share common characteristics: they approach AI scaling as a strategic business initiative rather than a technology project; they invest in the foundational capabilities needed for enterprise AI; and they recognize that organizational readiness is as critical as technical performance.
As AI continues to transform industries, the ability to move beyond isolated experiments to scaled implementation will increasingly separate market leaders from laggards. The roadmap outlined here provides a framework for organizations at any stage of the AI journey to accelerate their transition from promising pilots to production systems that deliver measurable business value.
What high-potential AI pilots in your organization are currently stuck in purgatory? And which of the scaling barriers outlined here resonates most with your experience? The answers to these questions may reveal your next opportunity for competitive advantage.